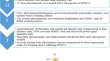
Overview
In Silico Pharmacology is an interdisciplinary journal focusing on computational approaches in drug design and multiscale analysis of bioactive substances.
- Bridges the gap between theory and experimental studies in ligand-target interactions and associated drug discovery and development.
- Publishes original research articles covering various aspects of pharmacology, drug discovery, and drug development, incorporating computational modelling and simulation.
- Highlights the advancement of cutting-edge molecular modelling, multiscale modelling, informatics, artificial intelligence, and big data methods in drug discovery and development.
- Welcomes state-of-the-art reviews at the forefront of computational methods intersecting with pharmaceutical research.
- Prioritizes papers with strong computational content or adequate experimental validation.
- Editor-in-Chief
-
- Boris Gutkin,
- Pramod Nair,
- Chang-Guo Zhan
- Submission to first decision (median)
- 11 days
- Downloads
- 101,421 (2023)
Latest articles
Journal updates
Journal information
- Electronic ISSN
- 2193-9616
- Abstracted and indexed in
-
- BIOSIS
- Baidu
- CLOCKSS
- CNKI
- CNPIEC
- Dimensions
- EBSCO
- EMBASE
- Google Scholar
- Japanese Science and Technology Agency (JST)
- Meta
- Naver
- OCLC WorldCat Discovery Service
- Portico
- ProQuest
- PubMedCentral
- Reaxys
- TD Net Discovery Service
- Wanfang
- Copyright information